AI Quant
Research Areas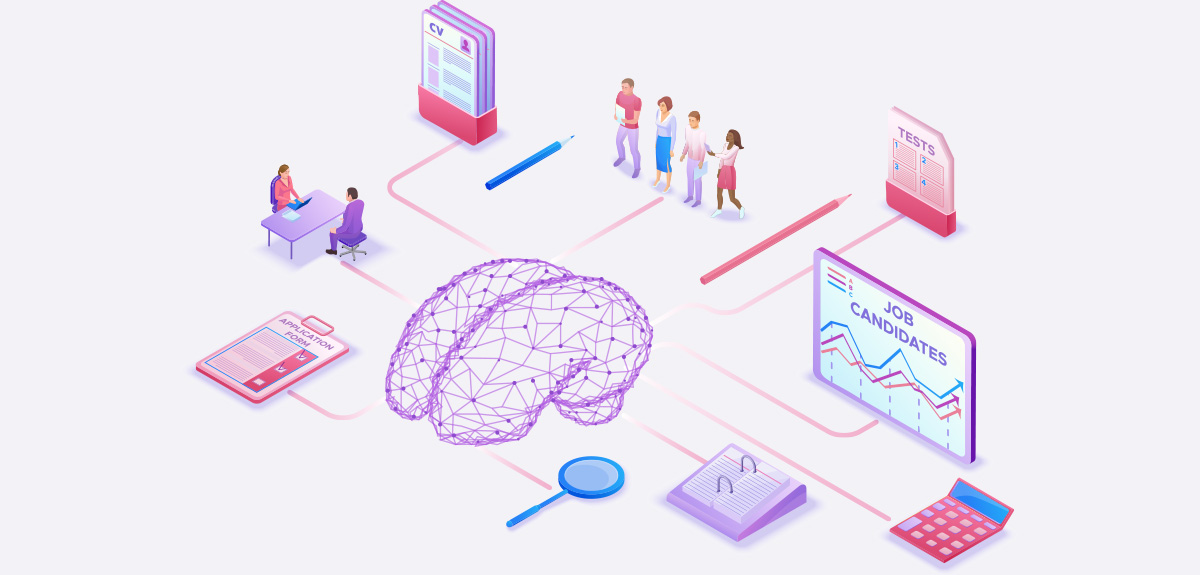
Intro
Humans sometimes make decisions and take action
according to emotion rather than logical reasoning. Wrong
decisions can come out of basing those decisions only on
one’s learning and experiences with data that is both biased
and partial. This is especially true of financial investments. A
person’s understanding of only the data that he or she knows
and emotional judgment lead to failed investments, which
often result in financial loss.
An area known as “Quant” is increasingly in the spotlight as a
mode of investment based on data, mathematical and
statistical methods that can potentially solve this problem.
Quant is short for “quantitative analyst” and refers to the
work of analyzing, predicting the financial markets or creating
an investment strategy based on mathematics and statistics.
The financial sector is experimenting with more applications
of recent radical developments in AI, with AI Quant emerging
as the most representative.
Thanks to the advancement of AI Quant, humans can process
even the wide range of data that they cannot perceive and
better understand and predict complicated financial markets.
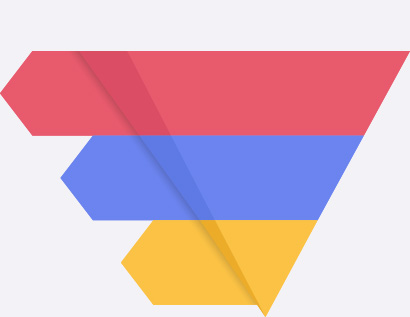
The Knowledge Funnel
- 01 Mystery
- 02 Heuristic
- 03 Algorithm
What is AI Quant?
The scope of AI Quant research is usually divided into four areas: data engineering,
quantitative investment algorithms, investment platforms and portfolios.
-
Data Engineering
- · Data Preprocessing
-
· Synthetic Data
Generation
- Structured Data
- Alternative Data
-
Algorithm
- · Alpha / Beta Strategy
- · Regime Detection
- · Portfolio Optimization
-
System
- · Infrastructure
- · Platform
- Backtesting System
- Parameter Optimization
-
Monitoring
& Planning - · Investor Profiling
- · Risk Management
- · Performance Evaluation
& Analysis - · Planning
Data engineering utilizes traditional financial and various alternative data to gather data faster and with higher
accuracy. Research on quantitative investment algorithms quickly and accurately backtests investment
algorithms based on a well-developed investment platform. It searches for statistically advantageous
situations and researches how investments can be made most effectively in these situations.
Besides risk monitoring for investors, research on investment platforms also provides investment performance
evaluation, while research on portfolios results in recommendations on the optimal portfolio make-up and
asset management for the customer. From an academic perspective, AI Quant stands at the intersection of
financial engineering and AI. It aims to provide better understanding of the financial markets through many
kinds of data and provide information and strategies that are as personalized as possible.
Future Research
HIT researches AI Quant technologies towards efficiently increasing customer assets.
Special focus is given to developing investment algorithms that produce robust profit
regardless of changes in economic circumstances, while minimizing customer losses.
We are also working on a personalized algorithm that recommends a portfolio most
suited to the investor’s current situation. Ultimately, our goal is to help customers build
up their assets and prepare for a financially-sound retirement.
In the future, the focal point of change will be the financial market’s ability, based on
objective figures earned through math and statistics, to increase the probability of
winning. HIT will continue to explore methods that have the highest potential for
effectively utilizing advantageous situations.